AI21 Labs, has just released Jamba, the world's first production-grade AI model based on the innovative Mamba architecture. Most models today (like GPT, Gemini and Llama) are based on the Transformer architecture. Jamba combines the strengths of both the Mamba Structured State Space model (SSM) and the traditional Transformer architecture, delivering impressive performance and efficiency gains.
Jamba boasts an extensive context window of 256K tokens, equivalent to around 210 pages of text, while fitting up to 140K tokens on a single 80GB GPU. This remarkable feat is achieved through its hybrid SSM-Transformer architecture, which leverages mixture-of-experts (MoE) layers to draw on just 12B of its available 52B parameters during inference. The result is a model that can handle significantly longer contexts than most of its counterparts, such as Meta's Llama 2 with its 32,000-token context window, while maintaining high throughput and efficiency.
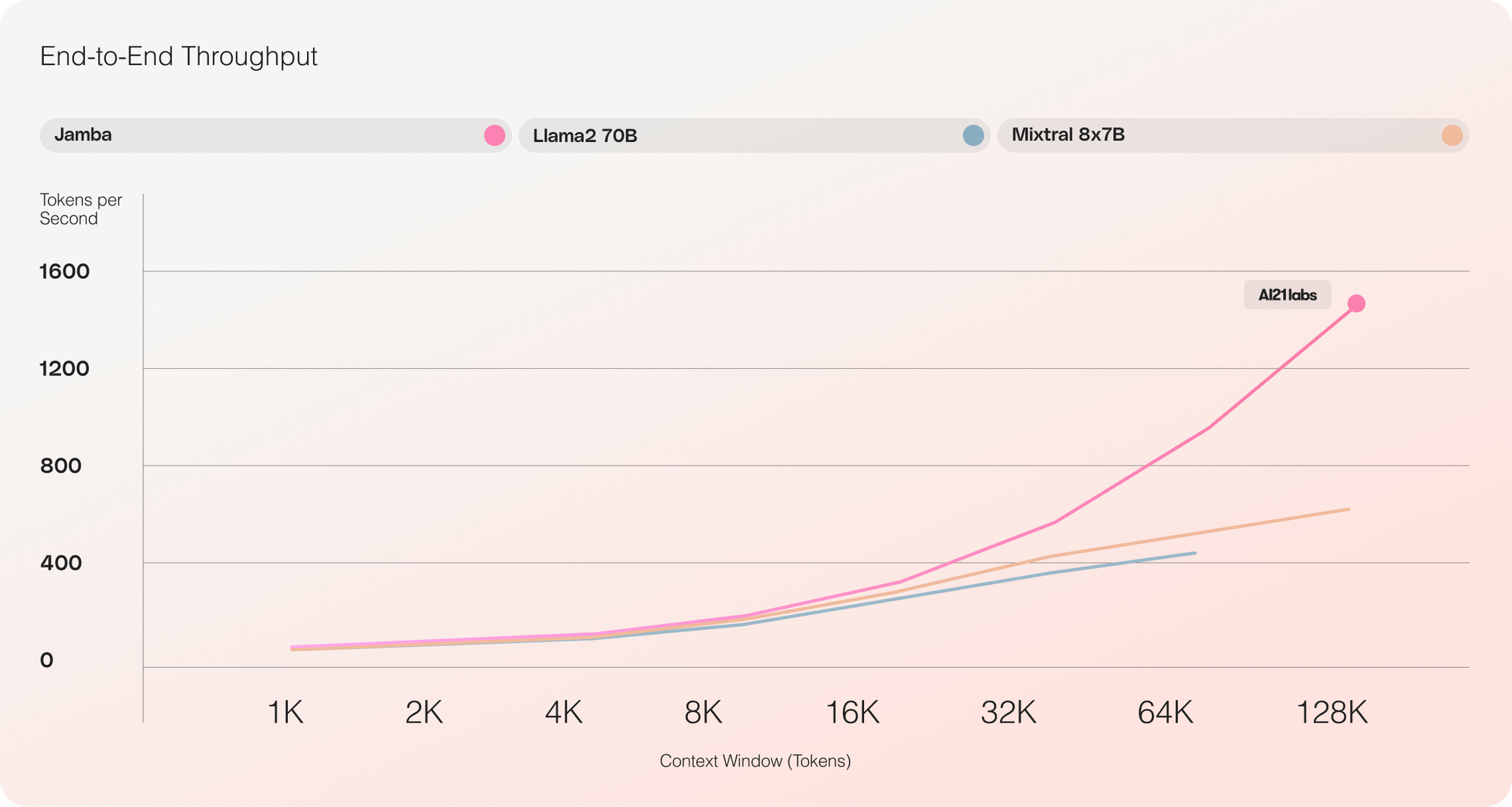
One of the key advantages of Jamba is its ability to deliver 3x throughput on long contexts compared to Transformer-based models of similar size, like Mixtral 8x7B. This is made possible by the model's unique hybrid architecture, which is composed of Transformer, Mamba, and mixture-of-experts (MoE) layers, optimizing for memory, throughput, and performance simultaneously.
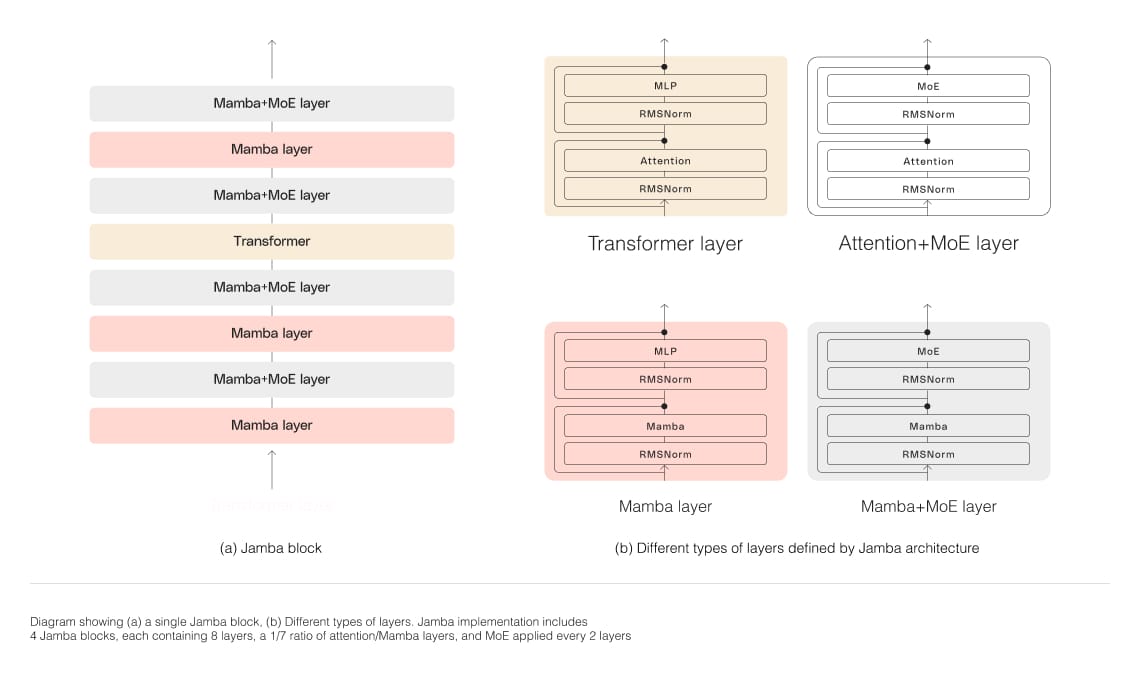
It features a blocks-and-layers approach, with each Jamba block containing either an attention or a Mamba layer, followed by a multi-layer perceptron (MLP). This results in an overall ratio of one Transformer layer out of every eight total layers. AI21 Labs says this approach allows the model to maximize quality and throughput on a single GPU, leaving ample memory for common inference workloads.
Jamba's impressive performance extends beyond efficiency and cost-effectiveness. The model has already demonstrated remarkable results on various benchmarks, matching or outperforming state-of-the-art models in its size class across a wide range of tasks.
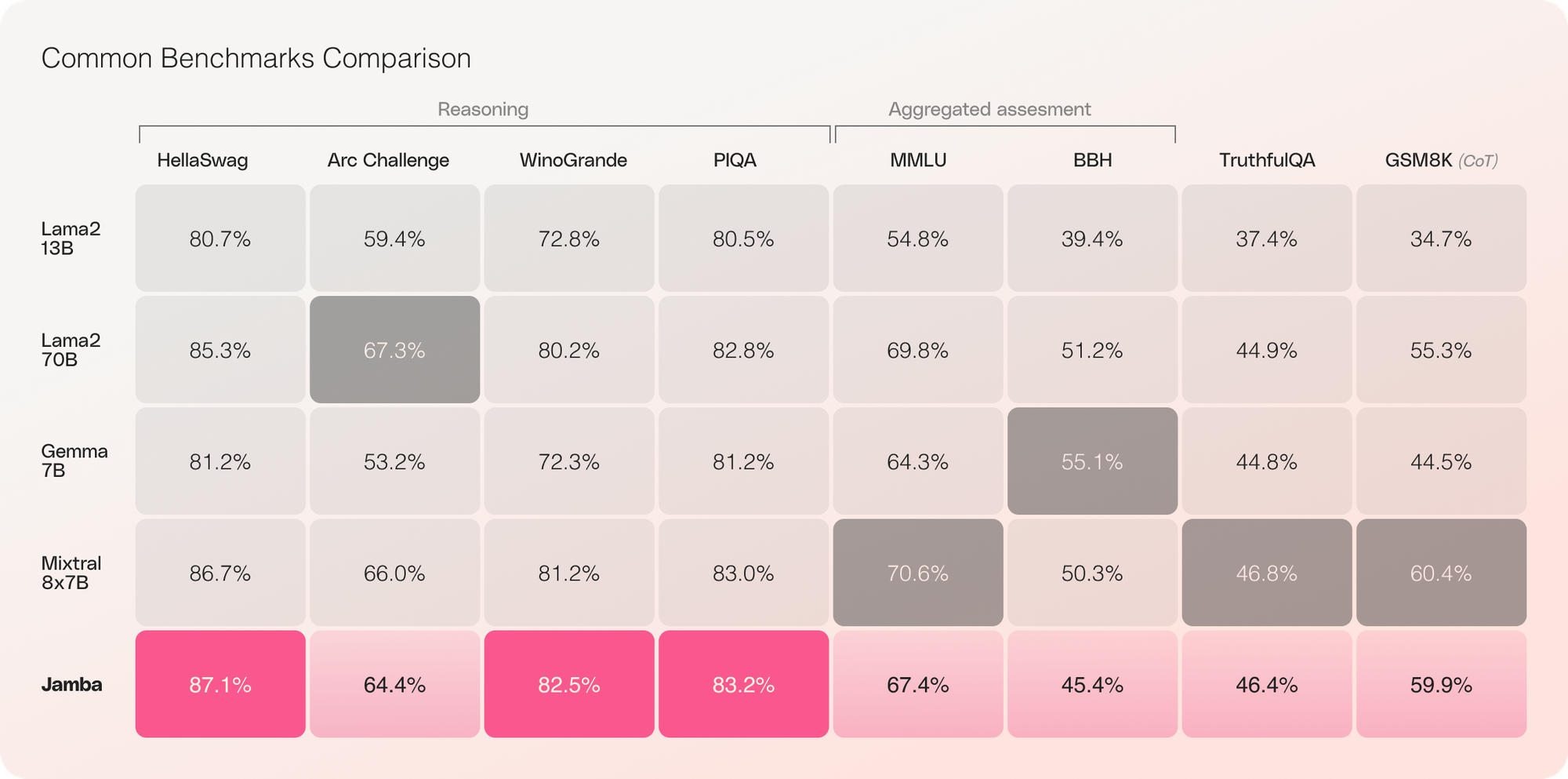
Jamba is being released with open weights under Apache 2.0 license. It is available on Hugging Face, and will also be accessible from the NVIDIA API catalog as NVIDIA NIM inference microservice, which enterprise applications developers can deploy with the NVIDIA AI Enterprise software platform.
For now, Jamba is currently released as a research model without the necessary safeguards for commercial use. However, AI21 Labs plans to release a fine-tuned, safer version in the coming weeks. As the AI community continues to explore and refine new architectures, we can expect to see even more impressive gains in performance, efficiency, and accessibility, paving the way for a new generation of more capable AI models.