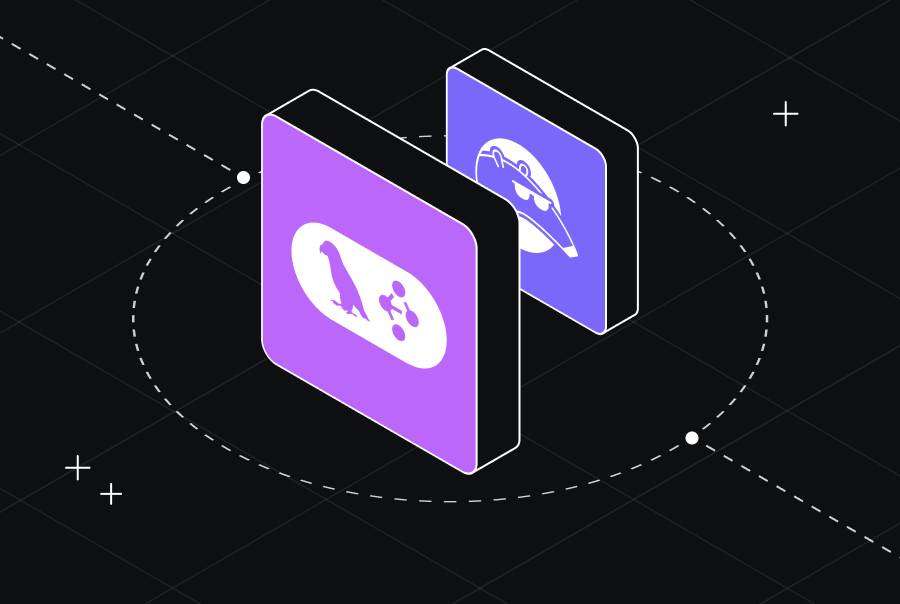
We’ve been building AI coding assistants at Qodo since the GPT-3 days. Our initial approach was highly structured with predefined flows for different coding tasks like test generation, code reviews, and improvements. This approach worked well with earlier generations of LLMs and with structured flows we were able to get real-world value from older models, despite all of their limitations.
Since Claude Sonnet 3.5 was released 9 months ago, LLMs have become significantly more capable at general-purpose coding tasks. The new models opened up the possibility to build something more dynamic and flexible while still maintaining our standards for code quality. We wanted to move away from rigid workflows to an agent that could adapt to any kind of user request, while still reflecting our opinionated views on how AI can be best used for coding.
Initially, we needed a framework that would let us quickly validate our ideas and from the few options that were available about 4 months ago, we settled on LangGraph for our initial proof of concept. We were pleasantly surprised to see that the framework has proven flexible and mature enough to carry us all the way to production.
In this post, I’ll explain why LangGraph was the right choice for us, and how it enabled us to build a coding assistant that balances flexibility with our opinionated approach to coding best practices.
Flexibility to be opinionated
Our key consideration was the ability to create opinionated workflows while maintaining adaptability. LangGraph takes a graph-based approach that gives you flexibility to build agents that land anywhere on the spectrum from completely open-ended — where you just give an LLM all available tools and let it run in a loop — to fully structured deterministic flows (like the ones we started with).
At its core, LangGraph lets you define a state machine for your agent. You create nodes that represent discrete steps in your workflow and edges that define the possible transitions between them. Each node can perform specific functions—gathering context, planning, generating code, or validating—while the graph structure determines how these functions connect.
The density of connections in the graph corresponds to how structured or flexible your agent is. A sparse graph with few connections corresponds with a more rigid, predictable flow where each step leads to exactly one next step. A dense graph with many interconnections gives the agent more freedom to choose its path.
Future, more capable models might work best with fully open-ended approaches. But even with the best current LLMs, you still get better results when you guide them through the problem. If you use LLMs directly for coding, you’ve probably already developed your own workflow — like breaking problems down, providing context strategically, guiding the model through complex reasoning, and backtracking or iterating when needed.
The nice thing about LangGraph’s flexibility is that we can easily recalibrate how structured our flows are when new, more powerful models are released.
Our main flow follows a pattern that you might recognize: first, a context collection node gathers relevant information from the codebase (and external resources via MCP integration); next, a planning node breaks down the task into manageable steps; then an execution node generates the actual code; finally, a validation node checks the output against best practices and requirements. When validation fails, the agent loops back to execution with specific feedback rather than starting from scratch.
Coherent interface
When you’re building a complex system, a framework should simplify rather than complicate your work. LangGraph’s API does exactly that.
Here’s how a simplified version of our main workflow looks when implemented with LangGraph:
from langgraph.graph import StateGraph, END workflow = StateGraph(name="coding_assistant") workflow.add_node("context_collector", collect_relevant_context) workflow.add_node("task_planner", create_execution_plan) workflow.add_node("task_executor", execute_plan) workflow.add_node("validator", validate_output) # Define flow between nodes workflow.add_edge("context_collector", "task_planner") workflow.add_edge("task_planner", "task_executor") workflow.add_edge("task_executor", "validator") # Conditional routing based on validation results workflow.add_conditional_edges( "validator", should_revise, { True: "task_executor", # Loop back if revision needed False: END # Complete if validation passes } ) graph = workflow.compile() graph.invoke({"user_input": "build me a game like levelsio"})
This declarative approach makes the code almost self-documenting. The workflow definition directly mirrors our conceptual diagram, which makes it easy to reason about and modify.
Each node function receives the current state and returns updates to that state. There’s no magic happening behind the scenes, just straightforward state transitions.
LangChain gets a lot of flack for its overly complicated abstraction, but the team really cooked with the LangGraph interface. It adds just enough structure without getting in your way or forcing you to adopt a complicated mental model and puts your agent logic on full display rather than obscuring it behind abstractions.
Reusable components across workflows
Reusability is what separates valuable frameworks from disposable ones. The node-based architecture that LangGraph uses is great here.
Our context collection node is a good example. It handles gathering relevant information from the codebase and it’s used in pretty much every flow. The same goes for our validation node, which checks code quality and runs tests. These components can slot into different graphs with minimal configuration.
As we build out more flows, the velocity payout is huge. We’re building specialized flows like TDD that have different structures but reuse many of the same nodes, just connected in a different configuration with a few specialized components added in.
State management
The most satisfying part of adopting the right framework is when you get useful functionality out of the box. LangGraph’s built-in state management is a perfect example.
Adding persistence to our agent took just a few lines of code:
from langgraph.graph import StateGraph, END from langgraph.checkpoint.postgres import PostgresSaver workflow = StateGraph(name="coding_assistant") ... ... checkpointer = PostgresSaver.from_conn_string( "postgresql://user:password@localhost:5432/db" ) checkpointer.setup() graph = workflow.compile(checkpointer=checkpointer)
That’s it. With this simple addition, our entire workflow state—including context collected, plans made, and code generated—persists to our postgres database without us building any custom infrastructure. There are also SQLite and in memory checkpointers that can be added just as easily.
What’s really neat is that this doesn’t just enable basic persistence across sessions. It supports checkpoints and branch points so you can undo and replay changes.
Areas for growth
While LangGraph has been a great foundation for our agentic flows, it’s not without challenges. One pain point has been documentation. The framework is developing very quickly and the docs are sometimes incomplete or out of date. The maintainers are great and were super responsive on Slack (thanks Harrison and Nuno for all the help :)). Be prepared to potentially need to communicate directly with the project maintainers if you’re using the newer and more niche capabilities.
Testing and mocking is a huge challenge when developing LLM driven systems that aren’t deterministic. Even relatively simple flows are extremely hard to reproduce. Our agent interacts extensively with the IDE, which is difficult to simulate in automated tests. We built a mock repository that simulates basic IDE operations, but it doesn’t perfectly replicate the real environment. This creates a gap between what we can test automatically and what happens in production.
For example, operations like “find all usages of this function” that depend on the IDE’s language server are particularly hard to mock. This forced us to rely more on manual testing than we’d prefer, which slowed down the iteration cycle.
Mature frameworks tend to provide robust infrastructure for mocking and testing. I’m hopeful that LangGraph will develop in these areas over time.